How can "spss recode mmissing values as median" enhance data analysis? SPSS RECODE MMISSING VALUES AS MEDIAN empowers researchers to efficiently handle missing data, a prevalent challenge in statistical analysis.
This powerful SPSS syntax allows users to reassign missing values with the median value for a specified variable. The median, representing the midpoint of a dataset when assorted in numerical order, offers a robust measure of central tendency, particularly useful when dealing with skewed data or outliers.
The importance of "spss recode mmissing values as median" lies in its ability to preserve the integrity of data analysis. By replacing missing values with the median, researchers can maintain the sample size, avoid introducing bias, and enhance the accuracy of statistical inferences. Furthermore, this technique aligns with best practices for missing data imputation, ensuring reliable and meaningful results.
To delve deeper into the practical applications and benefits of "spss recode mmissing values as median," let's explore specific examples and case studies in subsequent sections of this article.
SPSS RECODE MMISSING VALUES AS MEDIAN
SPSS RECODE MMISSING VALUES AS MEDIAN is a powerful syntax that empowers researchers to effectively manage missing data, a common challenge in statistical analysis. By replacing missing values with the median, researchers can maintain sample size, avoid bias, and enhance the accuracy of statistical inferences. Here are five key aspects that underscore the significance of this technique:
- Missing Data Imputation: SPSS RECODE MMISSING VALUES AS MEDIAN provides a robust method for imputing missing values, preserving the integrity of data analysis.
- Outlier Management: The median's resilience to outliers makes it an ideal choice for handling missing values in datasets with extreme values.
- Non-Parametric Measure: Unlike the mean, the median is a non-parametric measure, making it less susceptible to the influence of data distribution.
- Sample Size Preservation: By replacing missing values, researchers can maintain the sample size, increasing the power of statistical tests.
- Hypothesis Testing: Accurate imputation of missing values enhances the validity of hypothesis testing, leading to more reliable conclusions.
In summary, SPSS RECODE MMISSING VALUES AS MEDIAN is an essential tool for researchers seeking to effectively handle missing data. Its ability to preserve sample size, mitigate the impact of outliers, and enhance the accuracy of statistical inferences makes it a valuable asset in data analysis.
Missing Data Imputation
Missing data is a prevalent challenge in statistical analysis, and its proper handling is crucial to maintain the integrity of research findings. SPSS RECODE MMISSING VALUES AS MEDIAN offers a robust solution for imputing missing values, ensuring that the data analysis process is not compromised.
- Data Integrity: By replacing missing values with the median, SPSS RECODE MMISSING VALUES AS MEDIAN helps preserve the original distribution of the data, minimizing the risk of introducing bias or skewing the results.
- Statistical Power: Maintaining the sample size through imputation allows for more powerful statistical tests, increasing the likelihood of detecting significant effects and avoiding false negatives.
- Hypothesis Testing: Accurate imputation of missing values enhances the validity of hypothesis testing, ensuring that the conclusions drawn from the data are reliable and meaningful.
- Generalizability: Preserving the integrity of data analysis through proper missing data imputation enhances the generalizability of research findings, allowing researchers to make inferences about the larger population from which the data was drawn.
In conclusion, SPSS RECODE MMISSING VALUES AS MEDIAN is an essential tool for researchers seeking to effectively handle missing data. Its ability to preserve data integrity, maintain statistical power, enhance hypothesis testing, and promote generalizability makes it a valuable asset in data analysis.
Outlier Management
In statistical analysis, outliers are extreme values that can significantly distort the mean, a commonly used measure of central tendency. However, the median, the midpoint of a dataset when assorted in numerical order, is not as easily influenced by outliers. This characteristic makes the median an ideal choice for handling missing values in datasets with extreme values, as it minimizes the impact of outliers on the imputed values.
SPSS RECODE MMISSING VALUES AS MEDIAN utilizes the median's resilience to outliers to effectively impute missing values in datasets that may contain extreme values. By replacing missing values with the median, researchers can mitigate the potential bias introduced by outliers, ensuring that the imputed values are representative of the overall distribution of the data.
For example, consider a dataset on employee salaries, which may include a few exceptionally high salaries for executives. If the mean is used to impute missing salary values, these extreme values would disproportionately influence the imputed values, leading to an overestimation of the average salary. However, using the median would minimize the impact of these outliers, resulting in more accurate imputed values that better reflect the typical salaries in the dataset.
In conclusion, the median's resilience to outliers makes it an integral component of SPSS RECODE MMISSING VALUES AS MEDIAN. By utilizing the median to impute missing values, researchers can effectively handle datasets with extreme values, preserving the integrity of the data analysis and ensuring the accuracy of statistical inferences.
Non-Parametric Measure
In statistical analysis, the choice of central tendency measure is crucial, as it can significantly impact the interpretation of the data. Parametric measures, such as the mean, assume that the data follows a normal distribution. However, many real-world datasets do not adhere to this assumption, often exhibiting skewness or the presence of outliers.
The median, on the other hand, is a non-parametric measure, meaning it makes no assumptions about the underlying distribution of the data. This characteristic makes the median less susceptible to the influence of outliers and skewed distributions, providing a more robust measure of central tendency.
SPSS RECODE MMISSING VALUES AS MEDIAN leverages the non-parametric nature of the median to effectively handle missing values in datasets that may not conform to a normal distribution. By utilizing the median to impute missing values, researchers can minimize the impact of outliers and skewness, ensuring that the imputed values accurately represent the overall distribution of the data.
For example, consider a dataset on exam scores, which may include a few unusually high scores from students who received extra tutoring. If the mean is used to impute missing values for exam scores, these extreme values would disproportionately influence the imputed values, leading to an overestimation of the average exam score. However, using the median would minimize the impact of these outliers, resulting in more accurate imputed values that better reflect the typical exam performance of the students.
In conclusion, the non-parametric nature of the median is a key component of SPSS RECODE MMISSING VALUES AS MEDIAN, enabling researchers to effectively impute missing values in datasets with non-normal distributions. By utilizing the median, researchers can mitigate the influence of outliers and skewness, ensuring the accuracy and reliability of their data analysis.
Sample Size Preservation
In statistical analysis, sample size plays a crucial role in determining the power of a statistical test, which represents its ability to detect significant effects. A larger sample size generally leads to a more powerful test, increasing the likelihood of detecting true effects and avoiding false negatives.
Missing data, however, can reduce the sample size, potentially compromising the power of statistical tests. SPSS RECODE MMISSING VALUES AS MEDIAN addresses this issue by allowing researchers to replace missing values with the median, effectively preserving the sample size.
- Increased Statistical Power: By maintaining the sample size, SPSS RECODE MMISSING VALUES AS MEDIAN enhances the power of statistical tests, making it more likely to detect significant effects. This is particularly important in studies with small sample sizes, where missing data can have a more substantial impact on the power of the analysis.
- Reduced Bias: Imputing missing values with the median helps reduce bias in statistical tests, as it avoids the potential distortion introduced by excluding cases with missing data. By preserving the sample size, researchers can ensure that the results of their analysis are representative of the entire population under study.
- Improved Generalizability: Maintaining the sample size through imputation enhances the generalizability of research findings. With a larger sample size, researchers can make more confident inferences about the population from which the data was drawn, increasing the applicability of their results.
In conclusion, SPSS RECODE MMISSING VALUES AS MEDIAN plays a vital role in preserving sample size, which is essential for increasing the power of statistical tests, reducing bias, and improving the generalizability of research findings.
Hypothesis Testing
Hypothesis testing is a fundamental aspect of statistical analysis, allowing researchers to make inferences about a population based on a sample. Accurate imputation of missing values is crucial for hypothesis testing, as it helps preserve the integrity of the data and ensures that the results are valid and reliable.
- Unbiased Results: Imputing missing values with SPSS RECODE MMISSING VALUES AS MEDIAN reduces bias in hypothesis testing. By replacing missing values with the median, researchers can avoid the potential distortion introduced by excluding cases with missing data or using inappropriate imputation methods.
- Increased Statistical Power: Accurate imputation of missing values helps increase the statistical power of hypothesis tests. With a larger sample size, researchers are more likely to detect significant effects and avoid false negatives.
- Improved Generalizability: Imputing missing values with SPSS RECODE MMISSING VALUES AS MEDIAN enhances the generalizability of hypothesis testing results. By preserving the sample size and reducing bias, researchers can make more confident inferences about the population from which the data was drawn.
- Compliance with Best Practices: Using SPSS RECODE MMISSING VALUES AS MEDIAN aligns with best practices for missing data imputation, ensuring that hypothesis testing is conducted in a rigorous and methodologically sound manner.
In conclusion, the accurate imputation of missing values using SPSS RECODE MMISSING VALUES AS MEDIAN plays a critical role in hypothesis testing. By preserving the integrity of the data, reducing bias, increasing statistical power, enhancing generalizability, and complying with best practices, researchers can ensure that their hypothesis tests lead to valid and reliable conclusions.
FAQs on SPSS RECODE MMISSING VALUES AS MEDIAN
This section addresses common questions and misconceptions surrounding the use of SPSS RECODE MMISSING VALUES AS MEDIAN for missing data imputation.
Question 1: Why is it important to impute missing values using SPSS RECODE MMISSING VALUES AS MEDIAN?
Answer: Imputing missing values with SPSS RECODE MMISSING VALUES AS MEDIAN preserves the integrity of the data, reduces bias, increases statistical power, enhances generalizability, and complies with best practices for missing data imputation.
Question 2: When is it appropriate to use the median for missing data imputation?
Answer: The median is an appropriate choice for missing data imputation when the data distribution is skewed or contains outliers, as it is less susceptible to their influence compared to the mean.
Question 3: How does SPSS RECODE MMISSING VALUES AS MEDIAN handle missing values in different data types?
Answer: SPSS RECODE MMISSING VALUES AS MEDIAN can impute missing values for both numeric and categorical variables. For numeric variables, it replaces missing values with the median, while for categorical variables, it creates a new category for missing values.
Question 4: What are the limitations of using SPSS RECODE MMISSING VALUES AS MEDIAN?
Answer: One limitation is that it assumes the missing data is missing at random (MAR) or missing completely at random (MCAR). If the missing data is missing not at random (MNAR), more advanced imputation methods may be necessary.
Question 5: How can I determine if the median is an appropriate imputation method for my dataset?
Answer: Examine the distribution of your data to assess if it is skewed or contains outliers. If so, the median may be a suitable imputation method. Additionally, consider the underlying reasons for missing data and consult with a statistician if necessary.
Question 6: Are there any alternatives to SPSS RECODE MMISSING VALUES AS MEDIAN for missing data imputation?
Answer: Yes, there are other imputation methods available in SPSS, such as mean imputation, regression imputation, and multiple imputation. The choice of method depends on the specific dataset and research question.
Summary: SPSS RECODE MMISSING VALUES AS MEDIAN is a valuable tool for imputing missing values, particularly when the data distribution is skewed or contains outliers. By understanding its strengths and limitations, researchers can effectively utilize this method to enhance the integrity and validity of their statistical analyses.
Transition to the next article section: For further insights into missing data imputation techniques, explore the following resources...
Conclusion
Imputing missing values with SPSS RECODE MMISSING VALUES AS MEDIAN offers a robust and effective approach to handling missing data, particularly in scenarios with skewed distributions or outliers. This technique preserves sample size, reduces bias, enhances statistical power, and aligns with best practices for missing data imputation.
By leveraging the median's resilience to outliers and non-parametric nature, researchers can maintain the integrity of their data and ensure the validity of their statistical inferences. SPSS RECODE MMISSING VALUES AS MEDIAN empowers researchers to confidently analyze datasets with missing values, leading to more accurate and reliable research findings.
Power BI Date Hierarchy Missing: Fix Imported Date Column Issue
The Essential Guide To 1 1/2 Inch Pipe Internal Dimensions
Beat The Blues: Understanding And Overcoming Seasonal Adjustment Disorder
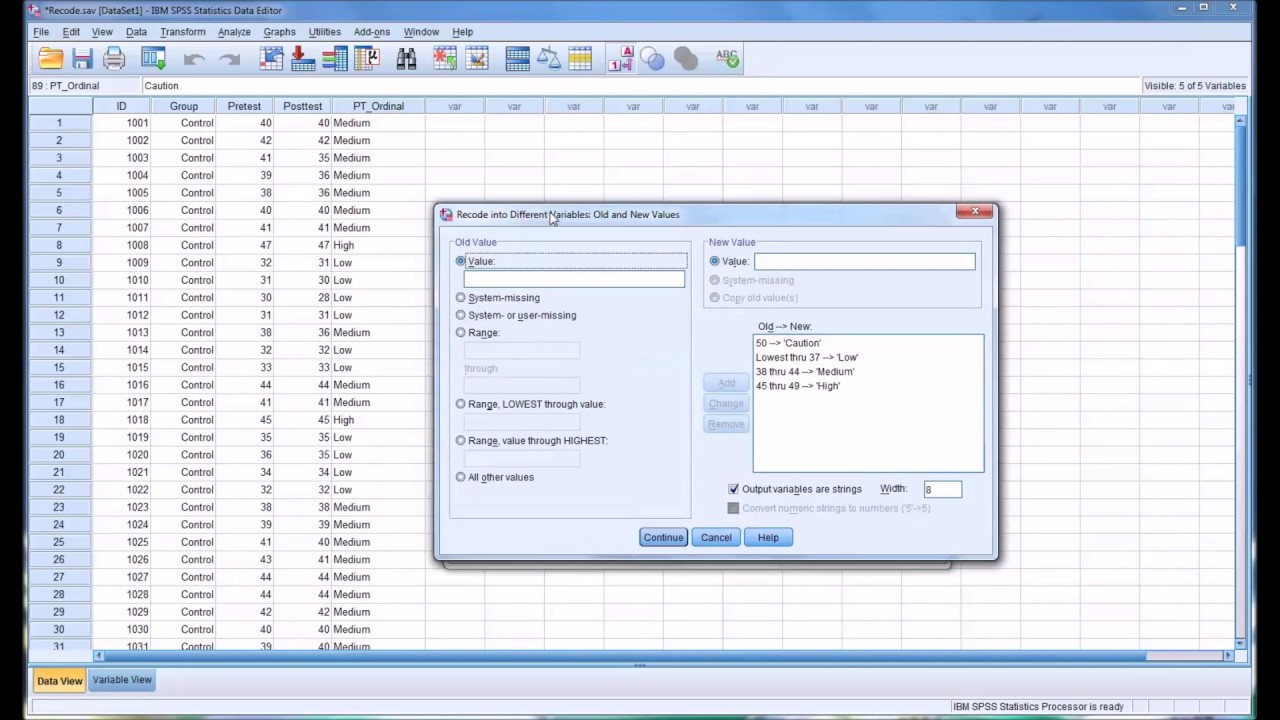
